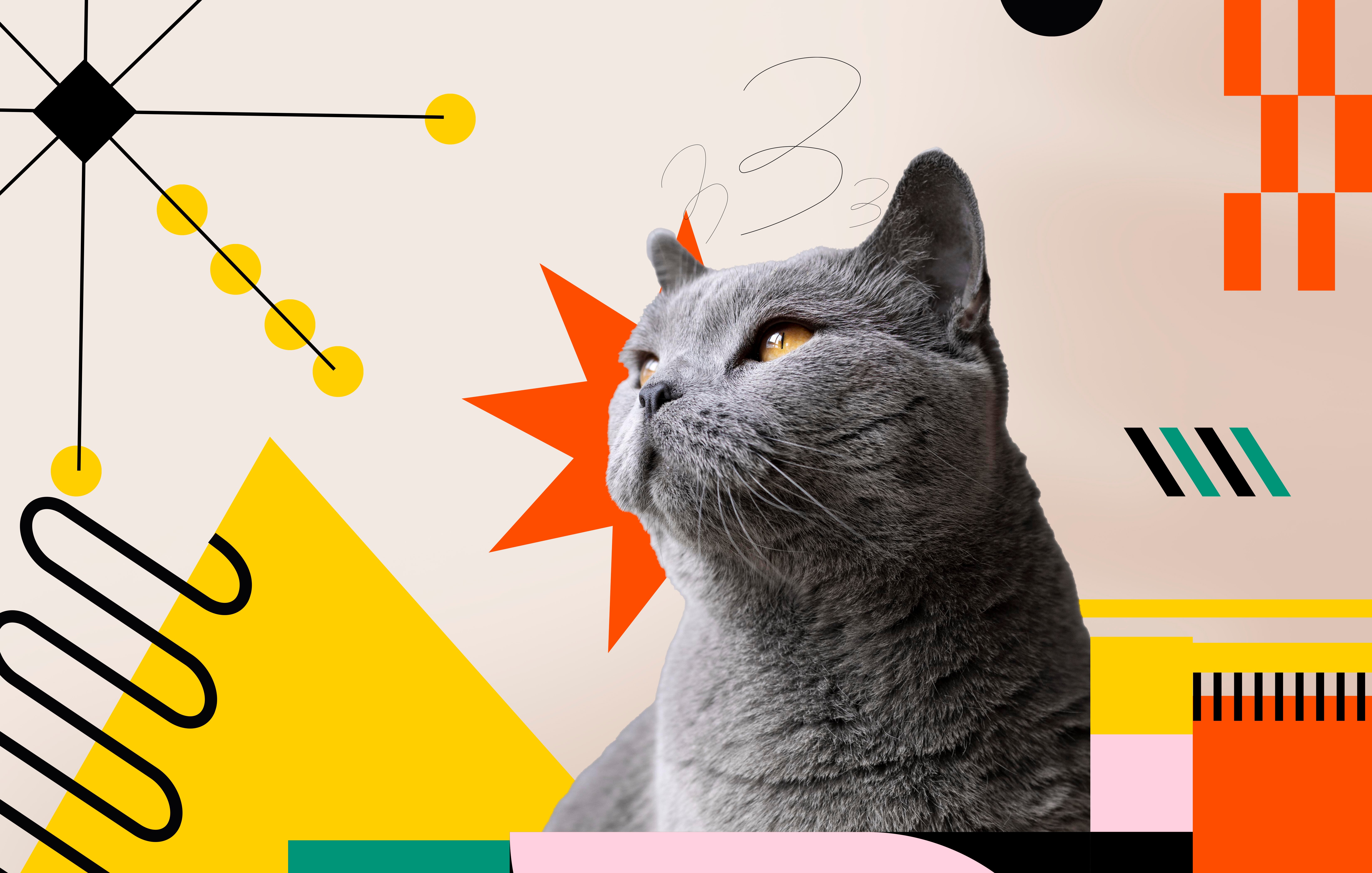
Bootstrapping is Here to Help (Including Cats to Help illustrate)
In research findings where random (probability) sampling was used, you will find a statement that specifies the margin of error as a measure of the accuracy or precision of the study results (“this survey has a margin of error of plus or minus X%, 19 times out of 20”). This statistic provides a straightforward indication of how closely a survey’s results reflect the actual population.
Two key assumptions underpin the the methods traditionally used in market and social research to estimate the margin of error and the standard error: 1) each individual in the sample was equally likely to be selected and 2) the measurements obtained from each individual were independent. In other words, simple random sampling was used to select the sample.
Most of the surveys we conduct in market and social research do not use a simple random sampling approach though. For example, in most studies we impose quotas (e.g., by region, age, and/or gender), which means that individuals in the population will have different probabilities of being selected. There is also the issue of non-response, which may or may not be random and could impact standard error estimation. Additionally, in some cases, for example with multistage probability sampling, the assumption of independence between measurements may not hold.
We generally assume that the traditional error estimation is sufficiently accurate. But what if we want to determine the accuracy of our estimations more precisely? In some cases, we may be able to use the information from the complex sample design to estimate sampling probabilities and adjust the standard errors so they are accurate, however this is usually very time consuming and requires extensive calculations. Also, this will still not account for non-response bias or lack of independence between observations.
Bootstrapping is a statistical procedure that provides an alternative to the traditional methods for calculating standard errors. Instead of taking multiple samples from a population to estimate the standard error, we create multiple samples from the original sample we collected. These samples are known as bootstrap samples. We create each of these bootstraps samples using simple random sampling with replacement, so each individual does have an equal chance of being selected.
Sampling designs generally require weighting to increase the representativeness of the sample, which adds a layer of complexity to conducting bootstrapping. By calculating and applying weights to each of the bootstraps samples we created, we can compute standard errors for different parameters of interest that are adjusted to be more representative of the population. One of the key advantages of using this method to estimate standard errors and variance is that, even if we do not have previous information about the sampling design, we can ensure that the analytical methods used are accurate. Another major benefit is that it is easier to implement and more flexible than most other methods available.
Bootstrapping illustrated1 Let's imagine we're interested in knowing the average weight of all the cats in Canada. Since it's not feasible to weigh every single cat, we randomly select a sample of 100 cats and weigh them. The average weight of these 100 cats is our sample mean, which we use as an estimate for the average weight of all Canadian cats. But we know that the sample mean could be influenced by which cats we happened to select. For instance, if we accidentally chose more overweight cats, our estimate might be too high. This is where bootstrapping comes in. In bootstrapping, we create a large number of "resamples" of your original sample, and calculate the statistic (in this case, the mean weight) for each resample. Each resample is created by drawing cats from our original sample with replacement. This means that after we select a cat to include in our resample, it goes back into the pool and could be selected again. Here's how a resample might work:
This is the core idea of bootstrapping: using resampling from our original sample to estimate the variability of a statistic. |
Advanis applied the bootstrapping approach on a recent study to understand if the year-over-year variation of the client’s satisfaction index was statistically significant. Using the bootstrap samples allowed Advanis to provide estimates of the level of accuracy of the index produced.
Late last year, Advanis also computed bootstrap samples, including weights, for the Public Health Agency of Canada (PHAC). In order to provide the PHAC with the ability to analyze a broad range of statistics, Advanis conducted bootstrapping and provided the PHAC with a total of 500 bootstrapped samples. Additionally, we weighted each bootstrapped sample to ensure that all replicates reflected a representative sample of Canadian parents/guardians, resulting in a total of 500 bootstrap weight variables.
We look forward to speaking to you about how bootstrapping can help improve the accuracy of your estimates for complex analysis and even more complex sampling plans.
1Adapted from ChatGPT. “Please explain statistical bootstrapping using a hypothetical situation with cats” prompt. ChatGPT, May 24 Version, OpenAI, June 13 2023, chat.openai.com.
Search / Recherche
Subscribe to our blog
Get our insights blog updates right to your mailbox once per month. No spam, we promise! Your email address is always safe.
Popular Tags
- ABpoll (1)
- Accessibility (2)
- Acedemics (1)
- Advertising (1)
- Analytics (3)
- API (1)
- Automation (1)
- Awareness (1)
- BC Food (1)
- Best Practices (8)
- BOS (1)
- Canada (7)
- Cannabis (2)
- Carbon Tax (1)
- Case Study (3)
- CATI (13)
- Choice (8)
- College (1)
- Communications (1)
- Concept Testing (2)
- Conference (4)
- Cordcutting (1)
- Covid-19 (2)
- CSAT (1)
- Customer Experience (12)
- Customer Journey (2)
- Dashboards (1)
- Data Science (2)
- Digital (1)
- Digital Adoption (1)
- DIY Research (2)
- Education (1)
- Email Marketing (1)
- Enviroment (1)
- Expectations (1)
- Finance (1)
- Foresight (2)
- Freelance (1)
- Future of Work (1)
- Gig Economy (1)
- Health (3)
- Human Experience (1)
- HX (1)
- Incentives (8)
- Innovation (5)
- Insights (22)
- Insurance (1)
- ISO (1)
- IVR (11)
- Learning (9)
- Longitudinal (1)
- Low Incidence Populations (2)
- Margin of Error (1)
- Market Research (34)
- Marketing (1)
- Marketing Primer (1)
- Millennial (8)
- Millennials (1)
- Mobile (17)
- MRX (21)
- newMR (1)
- NIS (1)
- NPS (10)
- Online Survey (5)
- Optimization (1)
- Persona (1)
- PMOT (1)
- Police Services (1)
- Project Management (1)
- Public Policy (3)
- Qualitative (10)
- Quant (2)
- Quantitative (10)
- Research (4)
- Response rates (4)
- Responsive (8)
- Sampling (5)
- Security (1)
- Segmentation (1)
- Social Research (3)
- Strategy (1)
- Success (4)
- Survey (9)
- Surveying (3)
- Tips and Tricks (2)
- Trends (8)
- University (1)
- USA (1)
- UX (1)
- Virtual (1)
- WCAG (1)
- Webinar (1)